TERRINet project SMILE
Structure-level Multi-sensor Indoor Localization Experiment
Global localization refers to situations when a map of the environment is known but there is no initial guess of the agent pose. Our PlaneLoc system integrates multiple local cues to construct a probability distribution that describes the likelihood of the agent pose. This framework enables to incorporate various types of localization cues, but as so far it was tested using segmented planes extracted from RGB-D data. We use multiple triplets of planar segments to generate candidate probability distribution and employ it to find the most probable pose with respect to a global map of planar segments. PlaneLoc evaluation on our own dataset (PUT RGB-D/Workshop) made it evident that the main limitation is the first generation RGB-D sensor, whose range was limited to approximately 4 m. Therefore, in the TERRINet TNA project we want to focus on passive cameras as data source for global indoor localization. However, passive sensors pose challenges regarding geometry recognition due to lack of direct depth information. Dense scene depth in passive stereo can be computed using classic algorithms, but results often inferior to human perception of the scene. As DNNs proven effective in learning when the structure of the underlying problem is not well understood, we plan to employ deep learning.
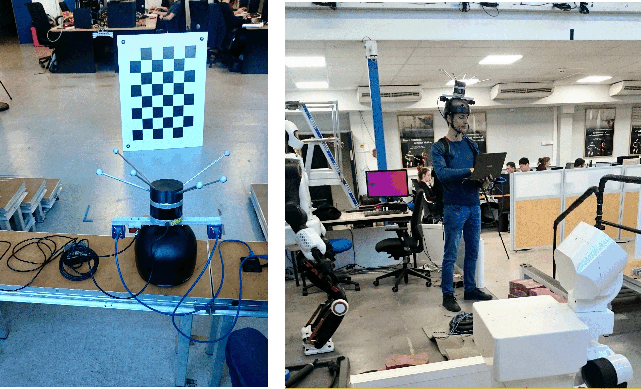
Our interest in the TERRINet TNA program stems from the need for data that can be used for development and tests of our indoor localization system. Since the deep learning „revolution” also robotics researches have realized that many problems related to interpretation of sensory data, features extraction and classification of features/objects can be solved applying the machine learning paradigm. Data interpolation methods that are learned from data tend to be more efficient and more general than their counterparts hand-crafted by human experts. hence, modern robotics became a sort of data science, and the availability of proper data for learning and evaluation became one of the main bottlenecks in research. this also applies to our PlaneLoc indoor localization system. Although we have successfully fielded a test version of PlaneLoc employing hand-crafted methods for the extraction of planar segments, we believe that scene description at the geometric structure-level is the main area for improvement in this system. Accordingly, we test machine learning-based algorithms for extraction and matching of geometric features: planar segments and edges. Unfortunately, we cannot find an existing dataset with the required, size, diversity, and ground truth for the further development of these methods. In this situation, the TERRINet TNA program brings an opportunity to explore the environment at LAAS-CNRS, which is an unique facility allowing to collect data of the required size and diversity of data, while providing the necessary accurate ground truth from a motion capture system. Our long-term goal is to improve the PlaneLoc system by enabling operation with passive vision systems (at first stereo, then also monocular), which will make it much more practical for personal localization, large-scale augmented reality applications, and service robots. A proper dataset for learning and evaluation plays an essential role in achieving this goal, and participation in the TERRINet should make these plans a reality.
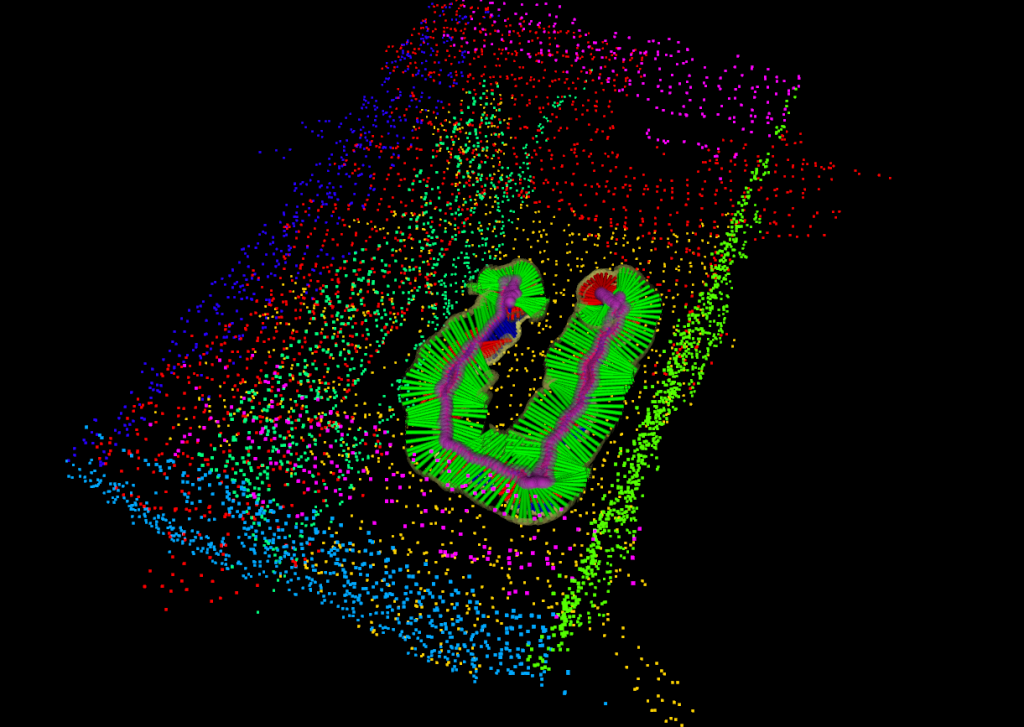
Inset image shows the corresponding images from the left camera
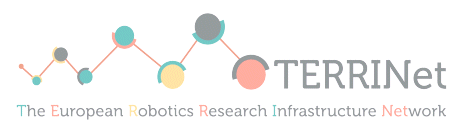